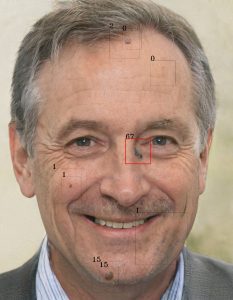
Model Dermatology has been validated in multiple academic studies conducted by prestigious university hospitals worldwide, with researchers from Korea, the United States, Chile, Greece, Australia, and Italy.
In experimental settings where the diagnosis was made solely with clinical photographs, the algorithm’s performance was comparable to that of dermatologists. For diagnosing suspected skin lesions, our multiclass algorithm’s performance was similar to that of dermatology residents in real-world settings. In Korea, we demonstrated the efficacy of augmented intelligence in a prospective randomized clinical trial.
However, in a melanoma study conducted in Italy, the algorithm's predictions did not align with those of dermatologists. Additionally, the algorithm that relies solely on photographs for diagnosis failed to match the accuracy of diagnoses made by actual dermatologists who incorporate various information.
Even so, in the 2022 Stiftung Warentest results from Germany, both the apps diagnosed by dermatologists and Model Dermatology showed nearly equal diagnostic rates. This indicates that while there are limitations to diagnosing solely based on photos, they still exhibit accuracy comparable to that of specialists under similar conditions.
The performance was comparable with that of dermatologists in the experimental setting.
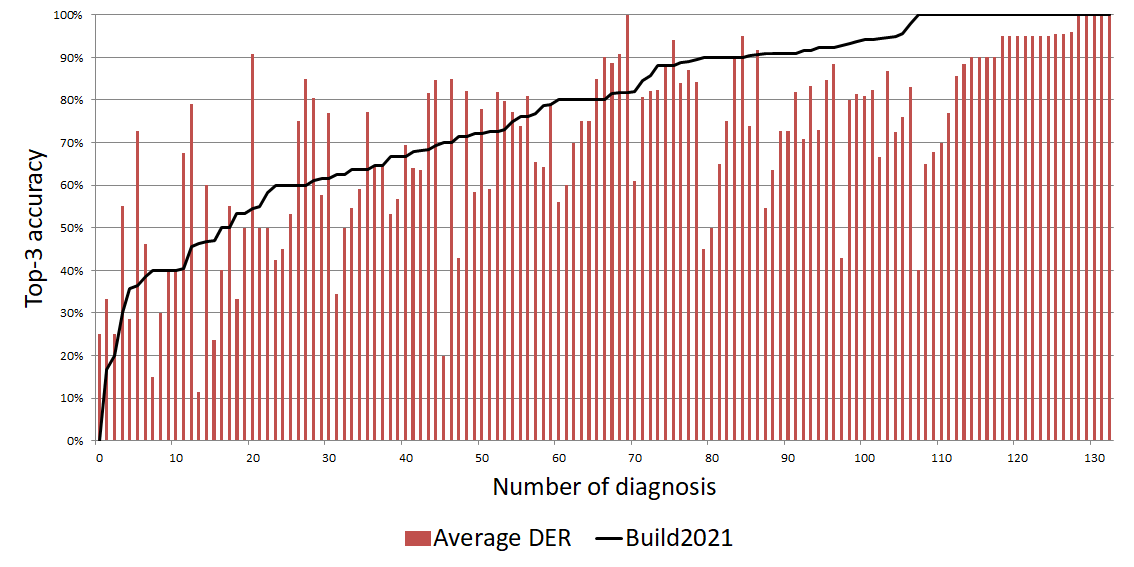
The algorithm could augment the performance of physicians in the real-world setting.
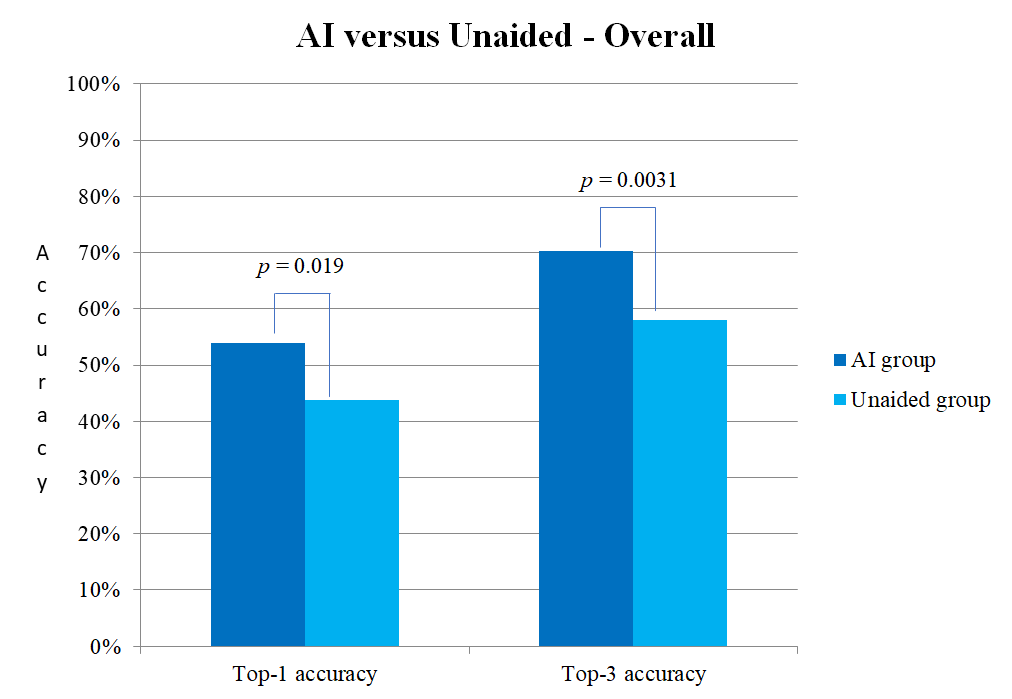
The algorithm could triage suspected skin lesions at the level of general physicians in the cohort validation using patient-captured images.
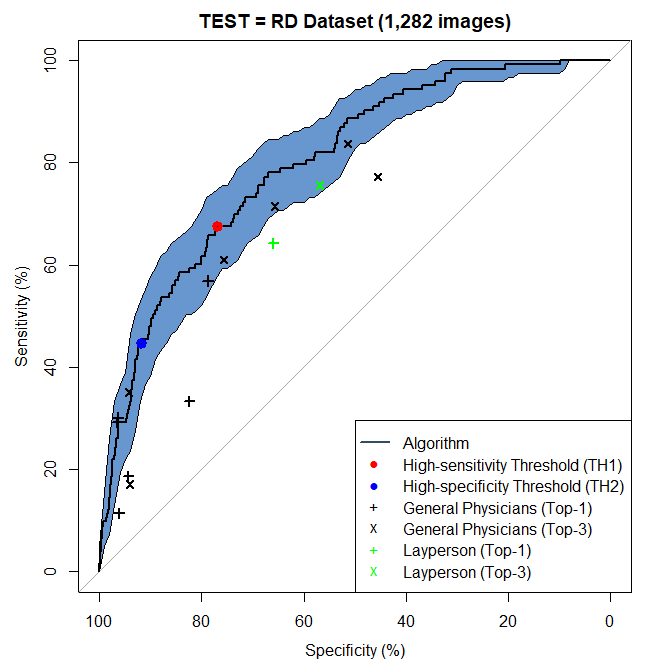
Clinical Study
- Assessment of Deep Neural Networks for the Diagnosis of Benign and Malignant Skin Neoplasms in Comparison with Dermatologists: A Retrospective Validation Study. PLOS Medicine, 2020
- Performance of a deep neural network in teledermatology: a single‐center prospective diagnostic study. J Eur Acad Dermatol Venereol. 2020
- Keratinocytic Skin Cancer Detection on the Face using Region-based Convolutional Neural Network. JAMA Dermatol. 2019
- Seems to be low, but is it really poor? : Need for Cohort and Comparative studies to Clarify Performance of Deep Neural Networks. J Invest Dermatol. 2020
- Multiclass Artificial Intelligence in Dermatology: Progress but Still Room for Improvement. J Invest Dermatol. 2020
- Augment Intelligence Dermatology : Deep Neural Networks Empower Medical Professionals in Diagnosing Skin Cancer and Predicting Treatment Options for 134 Skin Disorders. J Invest Dermatol. 2020
- Interpretation of the Outputs of Deep Learning Model trained with Skin Cancer Dataset. J Invest Dermatol. 2018
- Automated Dermatological Diagnosis: Hype or Reality? J Invest Dermatol. 2018
- Classification of the Clinical Images for Benign and Malignant Cutaneous Tumors Using a Deep Learning Algorithm. J Invest Dermatol. 2018
- Augmenting the Accuracy of Trainee Doctors in Diagnosing Skin Lesions Suspected of Skin Neoplasms in a Real-World Setting: A Prospective Controlled Before and After Study. PLOS One, 2022
- Evaluation of Artificial Intelligence-assisted Diagnosis of Skin Neoplasms – a single-center, paralleled, unmasked, randomized controlled trial. J Invest Dermatol. 2022
- The Degradation of Performance of a State-of-the-art Skin Image Classifier When Applied to Patient-driven Internet Search. Scientific Report 2022
- Generation of a melanoma and nevus data set from unstandardized clinical photographs on the internet. JAMA dermatology 2023
- Melanoma detection: Evaluating the classification performance of a deep convolutional neural network and dermatologist assessment via a mobile app in an Italian real-world setting. J Eur Acad Dermatol Venereol. 2024
Commentary
- Toward Augmented Intelligence: The First Prospective, Randomized Clinical Trial Assessing Clinician and Artificial Intelligence Collaboration in Dermatology – J Invest Dermatol. 2022
- Automated Classification of Skin Lesions: From Pixels to Practice – J. Invest Dermatol. 2018
- Problems and Potentials of Automated Object Detection for Skin Cancer Recognition – JAMA Dermatol. 2020
Magazine
- AI Beats Dermatologists in Diagnosing Nail Fungus (IEEE Spectrum, Feb. 2018)
The latest successful demonstration of AI’s capabilities in the medical field relied heavily upon a team of South Korean researchers putting together a huge dataset of almost 50,000 images of toenails and fingernails. That large amount of data used to train the deep neural networks on recognizing cases of onychomycosis—a common fungal infection that can make nails discolored and brittle—provided the crucial edge that enabled deep learning to outperform medical experts….
- New artificial intelligence system can empower medical professionals in diagnosing skin diseases (EurekAlert, March 2020)
Researchers in Korea have developed a deep learning-based artificial intelligence (AI) algorithm that can accurately classify cutaneous skin disorders, predict malignancy, suggest primary treatment options, and serve as an ancillary tool to enhance the diagnostic accuracy of clinicians…..
- AI-assisted diagnosis of skin neoplasms (Dermonaut, Oct 2022)
Short summary of the randomized controlled trial.
- Hautscreening-Apps (Stiftung Warentest, Dec 2022)
Stiftung Warentest was conducting an investigation into “skin screening apps”
Official document - https://www.test.de/Hautscreening-Apps-im-Test-5944602-0/
Full document is available here (Use the preview button to search for 264 pages) (https://books.google.co.kr/books?id=IzHnEAAAQBAJ ; page 264~266).
Blog
- AI in Medicine — Importance of Prospective Study (Medium, 2022)
It is essential that AI does well in the real-world setting. Furthermore, AI should be able to change the decision of doctors or patients. However, since the gap between the results of prospective and retrospective research is quite high, it is necessary to narrow down the scope of the problem, and we need to make a lot of effort to improve the data….
Contributors
Model Dermatology has been developed with the contribution of many academic researchers worldwide. Seung Seog Han (I Dermatology Clinic) is leading the project. Sung Eun Chang (Asan Medical Center), Jung-Im Na (Seoul National Univ.), Kyungho Paik (Seoul National Univ.), Seong Hwan Kim (Hallym Univ.), Myoung Shin Kim (Inje Univ.), Gyeong Hun Park (Hallym Univ.), Soo Ick Cho (Seoul National Univ.), Woohyung Lim (LG AI Research), Ik Jun Moon (Asan Medical Center), and Young jae Kim (Asan Medical Center) have contributed to the development of the algorithm since 2016 (1st author or senior author).
We are also grateful to Roxana Daneshjou (Stanford Univ.; USA), Justin Ko (Stanford Univ.; USA), Allan Halpern (Memorial Sloan Kettering Cancer Center; USA), Veronica Rotemberg (Memorial Sloan Kettering Cancer Center; USA), Ian Katz (Southern Sun Pathology; Australia), Fortunato Cassalia (Ospedale San Bortolo; Italy), Luigi Naldi (Ospedale San Bortolo; Italy), and Cristián Navarrete-Dechent (Pontificia Universidad Católica de Chile) who performed the external validation of the algorithm and provided commentary.
Mauricio Caceres (Paraguay) and Wail Atra (Sudan) have contributed to translation of the application.